
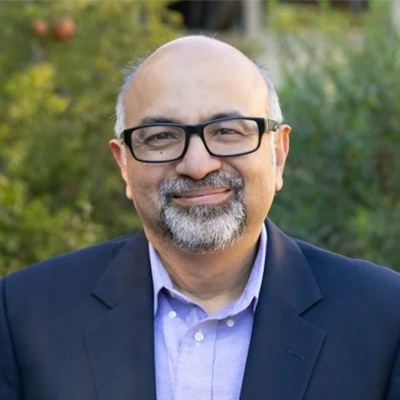
Dr. Sudipto Banerjee
Department(s)
BIOSTATISTICS
Office(s)
Office of the Dean
Title(s)
Professor and Chair of Biostatistics
Contact Info
Room 51-254B CHS
sudipto@ucla.edu
310.825.5916
Sudipto Banerjee, Ph.D., is a Professor in the Department of Biostatistics at the Fielding School of Public Health and in the Department of Statistics at the College of Physical Sciences with an affiliate appointment in the UCLA Institute of the Environment and Sustainability. His research expertise includes Bayesian hierarchical modeling and inference for complex systems involving massive datasets (“BIG DATA”); environmental processes and their impact on public health; spatial data science; spatial epidemiology; stochastic process models; statistical learning from physical and mechanistic systems; survey sampling and survival analysis. His theoretical and methodological contributions in statistics are available in the form of several scholarly publications in highly regarded peer-reviewed journals in statistics, biostatistics and health sciences; an authoritative textbook on spatial statistics; a textbook on linear algebra for statisticians; an edited comprehensive handbook on spatial epidemiology; and two committee reports for the National Research Council of the National Academies. He has served as Principal Investigator of over 14 major federally funded research projects from NIH and NSF primarily devoted to the advancement of statistical theories and methods for space-time processes and their substantive impact. Methods developed by Professor Banerjee are being widely employed in epidemiological and environmental health research to enhance scientific understanding of how environmental factors affect human health over space and time. He has made pioneering contributions in several important areas at the interface of spatial data science and health research including spatial survival analysis; space-time mapping and analysis of multiple diseases; and analyzing massive volumes of space-time data (BIG DATA analytics). He has played prominent roles in substantive public health projects such as “The GuLF Study” (Deepwater Horizon disaster) and is currently overseeing data analysis efforts to evaluate the health effects from the massive natural gas leak disaster in Aliso Canyon in California.
Professor Banerjee’s research and scholarship has been recognized with honors such as the Abdel El-Shaarawi Award from The International Environmetric Society (TIES), the Mortimer Spiegelman Award from the American Public Health Association and the George W. Snedecor Award from the Committee of Presidents of Statistical Societies (COPSS), elected membership of the International Statistical Institute, elected fellowships in the Institute of Mathematical Statistics (IMS), the American Statistical Association (ASA), the International Society for Bayesian Analysis (ISBA) and the American Association for the Advancement of Science (AAAS), a Distinguished Achievement Medal from the ASA’s Section on Statistics and the Environment, and the ASA’s Outstanding Statistical Application Award. He was elected to serve as President of the International Society for Bayesian Analysis (ISBA) in 2022 (President-Elect in 2021; Past-President in 2023).
Educational History
B.S. (Honours) Presidency College, Calcutta, India, 1994.
M.STAT. Indian Statistical Institute, Calcutta, India, 1996.
Ph.D. Statistics, University of Connecticut, Storrs, Connecticut, USA, 2000.
Research Interests
6 Overview of scholarly research
6.1 Multivariate spatial processes and applications:
6.2 Modeling and analysis for spatially-referenced survival data
6.3 Statistical inference for spatial gradients and identifying zones of rapid change
6.4 Modeling and inference for large, or massive, spatial-temporal data sets (“BIG DATA”)
6.5 Disease mapping and spatial boundary analysis
6.6 Bayesian modeling and statistical learning from mechanistic systems
6.7 Case studies requiring statistical innovation
6.8 Computational methods, algorithms and statistical software development
6.9 Impact and relevance of scholarly research
6.10 Textbooks and Monographs
Honors and awards
- 2005, Inductee: Pi Chapter of Delta Omega National Honor Society.
- 2009, Abdel El Sharaawi Young Researcher Award from The International Environmetrics Society.
- 2010, Elected member, International Statistical Institute.
- 2011, Mortimer Spiegelman Award from the Statistics Section of the American Public Health Association.
- 2012, Elected Fellow of the American Statistical Association (ASA).
- 2012, International Indian Statistical Association’s Young Researcher Award.
- 2015, Presidential Invited Address, Western North American Regional (WNAR) Meeting of the International Biometric Society.
- 2015, Elected Fellow of the Institute of Mathematical Statistics (IMS).
- 2015, Distinguished Achievement Medal from ASA Section on Statistics and the Environment.
- 2017, ASA Outstanding Application Award.
- 2018, Elected Fellow of the International Society for Bayesian Analysis (ISBA).
- 2019, George W. Snedecor Award from the Committee of Presidents of Statistical Societies (COPSS).
- 2020, Elected Fellow of the American Association for the Advancement of Science (AAAS).
- 2022, President of the International Society for Bayesian Analysis.
Books
Hierarchical Modeling and Analysis for Spatial Data. Second Edition
Keep up to date with the evolving landscape of space and space-time data analysis and modeling. Since the publication of the first edition, the statistical landscape has substantially changed for analyzing space and space-time data. More than twice the size of its predecessor, Hierarchical Modeling and Analysis for Spatial Data, Second Edition reflects the major growth in spatial statistics as both a research area and an area of application. → Details
Linear Algebra and Matrix Analysis for Statistics
Linear Algebra and Matrix Analysis for Statistics offers a gradual exposition to linear algebra without sacrificing the rigor of the subject. It presents both the vector space approach and the canonical forms in matrix theory. The book is as self-contained as possible, assuming no prior knowledge of linear algebra. Beginning with the rudimentary mechanics of linear systems, the book gradually develops a formal development of Euclidean vector spaces, rank and inverse of matrices, orthogonality, projections and projectors, eigenvalues, eigenvectors, different matrix decompositions (e.g., LU, Cholesky, QR, spectral, SVD, Jordan), positive definite matrices, Kronecker and Hadamard products, and concludes with accessible treatments of some more specialized topics, such as linear iterative systems, convergence of matrices, Markov chains and Page-Rank algorithms, and more general vector spaces. → Details
Handbook of Spatial Epidemiology
Handbook of Spatial Epidemiology explains how to model epidemiological problems and improve inference about disease etiology from a geographical perspective. Top epidemiologists, geographers, and statisticians share interdisciplinary viewpoints on analyzing spatial data and space–time variations in disease incidences. These analyses can provide important information that leads to better decision making in public health. → Details
Reports
National Academies: Affordability of National Flood Insurance Program Premiums
Affordability of National Flood Insurance Program Premiums: Report 1 is the first part of a two-part study to provide input as FEMA prepares their draft affordability framework. This report discusses the underlying definitions and methods for an affordability framework and the affordability concept and applications. Affordability of National Flood Insurance Program Premiums gives an overview of the demand for insurance and the history of the NFIP premium setting. The report then describes alternatives for determining when the premium increases resulting from Biggert-Waters 2012 would make flood insurance unaffordable. → Details
Featured publications over the last 5 years
Halder, A., Banerjee, S. and Dey, D.K. (in press). Bayesian modeling with spatial curvature processes. Journal of the American Statistical Association. arxiv and DOI.
Banerjee, S. (in press). Finite population survey sampling: An unapologetic Bayesian perspective. Sankhya A. arxiv and DOI
Li, D., Tang, W. and Banerjee, S. (2023). Inference for Gaussian Processes with Matern covariogram on compact Riemannian manifolds. Journal of Machine Learning Research, 24(101), 1–26. arxiv and Journal Link
Alaimo Di Loro, P., Mingione, M., Lipsitt, J., Batteate, C.M., Jerrett, M.B. and Banerjee, S. (2023). Bayesian hierarchical modeling and analysis for physical activity trajectories using wearable devices data. Annals of Applied Statistics, 17, 2865–2886. arxiv and DOI
Gao, L., Banerjee, S. and Ritz, B. (2023). Spatial difference boundary detection for multiple outcomes using Bayesian disease mapping. Biostatistics, 24, 922–944. arxiv and DOI.
Dey, D., Datta, A. and Banerjee, S. (2022). Graphical Gaussian process models for highly multivariate spatial data. Biometrika, 109, 993–1014. arxiv and DOI.
Banerjee, S. (2022). Discussion of “Measuring housing vitality from multi-source big data and machine learning”. Journal of the American Statistical Association, 117, 1063–1065. DOI.
Peruzzi, M., Banerjee, S. and Finley, A.O. (2022). Highly scalable Bayesian geostatistical modeling via meshed Gaussian Processes on partitioned domains. Journal of the American Statistical Association, 117, 969–982. arxiv and DOI.
Zhang, L. and Banerjee, S. (2022). Spatial factor modeling: A Bayesian Matrix-Normal approach for misaligned data. Biometrics, 78, 560–573. arxiv and DOI.
Tang, W., Zhang, L. and Banerjee, S. (2021). On identifiability and consistency of the nugget in Gaussian spatial process models. Journal of the Royal Statistical Society: Series B (Methodology), 83, 1044–1070. arxiv and DOI.
Abdalla, N., Banerjee, S., Ramachandran, G. and Arnold, S. (2020). Bayesian state space modeling of physical processes in industrial hygiene. Technometrics, 62, 147–160. arxiv and DOI.
Finley, A.O., Datta, A., Cook, B.C., Morton, D.C. Andersen, H.E. and Banerjee, S. (2019). Efficient algorithms for Bayesian nearest-neighbor Gaussian processes. Journal of Computational and Graphical Statistics, 28, 401–414. arxiv and DOI.