6 Overview of scholarly research
Professor Banerjee’s research contributions have focused on the development of statistical methods and Bayesian inferential frameworks for spatial-temporal data sets, exploring their theoretical properties, and their applications to substantive scientific fields. He has also published scholarly articles in other fields of statistics including, but not limited to, survival analysis; hierarchical state-space modeling for physical processes; Bayesian learning and calibration in mechanistic systems; Bayesian network models; statistical computing and software development; and Bayesian approaches finite population survey sampling methods. His collaborative work has focused on diverse scientific applications of Bayesian statistics in public health, the broader environmental sciences, climate science, ecology, forestry, social sciences and economics.
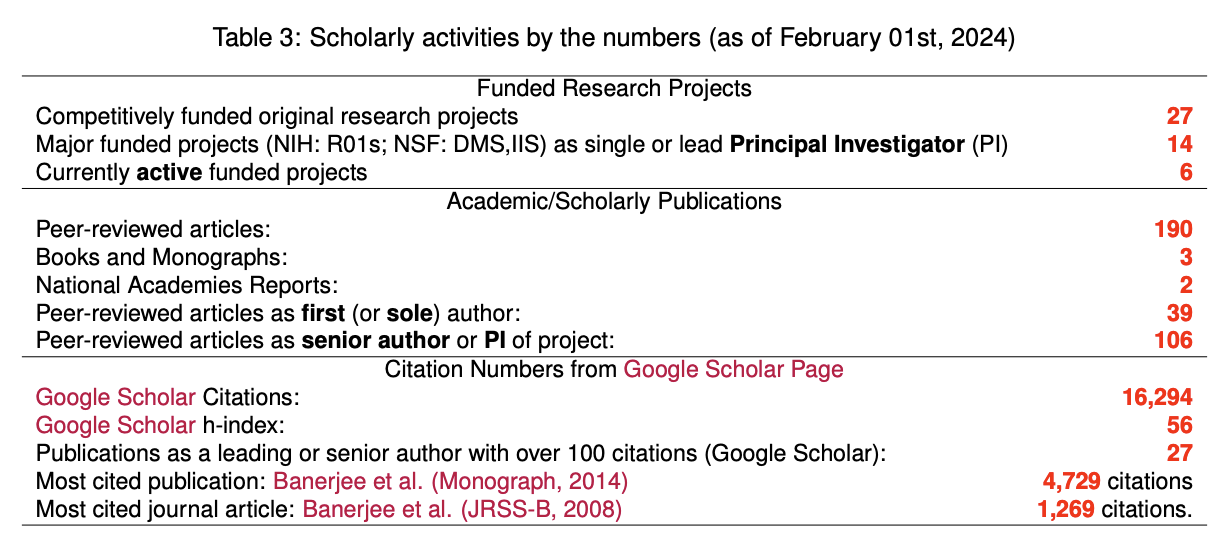
As of March 15th, 2023, Professor Banerjee has been the Principal Investigator (PI) of 14 major federally funded projects out of 27 projects with 6 currently active projects. Apart from multiple R01 awards from NIH as sole or lead PI, a particularly notable achievement of his in the space of securing competitive extramural funding is his being awarded an NIH Challenge Grant (RC1) as part of the American Recovery & Reinvestment Act (ARRA). Through this and his several other awards as a scholarly leader, Professor Banerjee pioneered statistical methodologies and computational algorithms for analyzing massive spatialtemporal databases that have had significant impact on diverse scientific issues surrounding climate and environmental data science and their impact on public health. Of his six currently active projects, Professor Banerjee is Principal Investigator on 3 R01s from NIH and 1 DMS grant from NSF. In addition, he is currently leading data analysis efforts in a significant USD 21 million project to evaluate the health effects from the major natural gas leak disaster in Aliso Canyon, CA, in 2015-2016. Professor Banerjee has published 175 peer-reviewed scholarly articles. He is the first author in 37 peer-reviewed publications (including 8 as solo author) and has assumed a leadership role in an additional 82 peer-reviewed publications through 10 Table 4: A selection of notable journals that have carried Professor Banerjee’s articles either as leading or senior author, the Principal Investigator (PI) funding the project or as the senior (or sole) statistician in a substantive collaboration. The journal names are active links to their websites containing information on their aims and scope, and citation metrics.
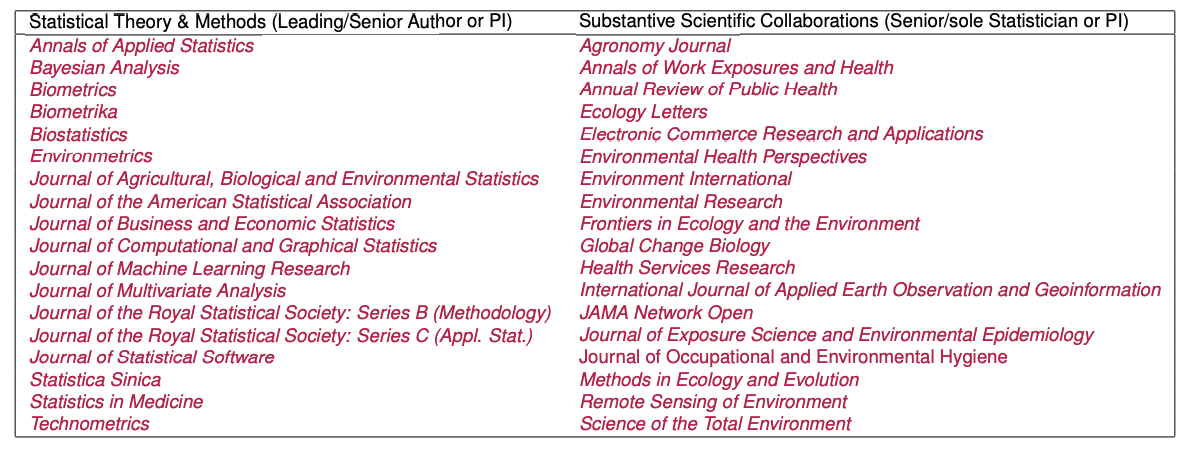
supervision of a doctoral dissertation with his student as the first author or as the Principal Investigator of a project funded by federal agencies, where the first author has been a current or former student, postdoctoral fellow, a junior colleague, or a graduate student. Table 3 presents a summary of Professor Banerjee’s career wide scholarly activities by the numbers. Notable scholarly journals where Professor Banerjee has published his scholarly research on developing statistical methods, exploring theoretical aspects of statistical models, and applying them to substantive scientific applications are included in Table 4. The left hand column lists a selection of notable journals that are focused on statistical theory and methods, while the right hand column presents a selection of journals focusing upon scientific explorations and discoveries. Professor Banerjee’s research leadership is manifested through his pioneering and foundational contributions to statistical theory and methods for analyzing spatially oriented data with applications in the natural and environmental sciences, and in public health. While a comprehensive review of his scholarly manuscripts is beyond the scope of a single letter, some of his key contributions to statistical science are briefly described below based upon different topics. Five key peer-reviewed publications with Professor Banerjee as either the leading or a senior author or PI funding the research are selected for each topic. The Digital Object Identifier (DOI) for each publication is provided as active links to the articles.
6.1 Multivariate spatial processes and applications:
Multivariate spatial processes refer to collections of spatial or spatial-temporal processes, where each process captures spatial dependence while also being associated with the other processes in the collection. Building valid multivariate processes and multivariate spatial probability laws is a crucial exercise in the analysis of environmental and public health data where different spatially-oriented variables of interest are associated among themselves in addition to being spatially dependent. Multivariate processes also arise in constructing spatially varying regression models, where the slopes in the regression model are spatial processes designed to capture associations in the way they impact the outcome or response over space and time. Statistical models for capturing multivariate spatial dependencies must also overcome complications arising from spatial misalignment and change of support problems, which refer to settings when not all of the variables of interest have been measured over the same set of spatial locations. Professor Banerjee has published an array of articles on developing rich and flexible yet computationally practicable 11 methods for analyzing complex multivariate dependencies in spatial data arising in environmental sciences and public health research. Five important publications on different aspects of this research include:
1. Banerjee, S. and Johnson, G.A. (2006). Coregionalized single and multi-resolution spatially varying growth curve modeling with application to weed growth. Biometrics, 62, 864–876. DOI: http://dx. doi.org/10.1111/j.1541-0420.2006.00535.x
2. Jin, X., Banerjee, S. and Carlin, B.P. (2007). Order-free coregionalized areal models with application to multiple disease mapping. Journal of the Royal Statistical Society: Series B (Methodology), 69, 817–838. DOI: http://dx.doi.org/10.1111/j.1467-9868.2007.00612.x
3. Banerjee, S., Finley, A.O., Waldmann, P. and Ericsson, T. (2010). Hierarchical spatial process models for multiple traits in large genetic trials. Journal of the American Statistical Association, 105, 506–521. DOI: http://dx.doi.org/10.1198/jasa.2009.ap09068
4. Zhang, L. and Banerjee, S. (2022). Spatial factor modeling: A Bayesian Matrix-Normal approach for misaligned data. Biometrics, 78, 560–573. DOI: https://doi.org/10.1111/biom.13452.
5. Dey, D., Datta, A. and Banerjee, S. (2022). Graphical Gaussian process models for highly multivariate spatial data. Biometrika, 109, 993–1014. DOI: https://doi.org/10.1093/biomet/asab061.
6.2 Modeling and analysis for spatially-referenced survival data:
Spatial variation in survival patterns often reveal underlying lurking factors, which, in turn, assist researchers and data science professionals in their decision making process to identify regions requiring attention. Dr. Banerjee pioneered the development of hierarchical models for spatially dependent time-to-event data. The following is a list of five publications relevant to spatial survival analysis:
1. Banerjee, S., Wall, M. and Carlin, B.P. (2003). Frailty modeling for spatially correlated survival data with application to infant mortality in Minnesota. Biostatistics, 4, 123–142. DOI: http://dx.doi.org/ 10.1093/biostatistics/4.1.123
2. Banerjee, S. and Carlin, B.P. (2004). Parametric spatial cure rate models for interval-censored time to relapse data. Biometrics, 60, 268–275. DOI: http://dx.doi.org/10.1111/j.0006-341X.2004. 00032.x
3. Cooner, F., Banerjee, S., Carlin, B.P. and Sinha, D. (2007). Flexible cure rate modeling under latent activation schemes. Journal of the American Statistical Association, 102, 560–572. DOI: http: //dx.doi.org/10.1198/016214507000000112
4. Banerjee, S., Kauffman, R.J. and Wang, B. (2007). Modeling Internet firm survival using Bayesian dynamic models with time-varying coefficients Electronic Commerce Research and its Applications, 6, 332–342. DOI: http://dx.doi.org/10.1016/j.elerap.2006.06.004
5. Diva, U., Dey, D.K. and Banerjee, S. (2008). Parametric models for spatially correlated survival data for individuals with multiple cancers. Statistics in Medicine, 27, 2127–2144. DOI: http://dx.doi. org/10.1002/sim.3141
6.3 Statistical inference for spatial gradients and identifying zones of rapid change:
Stochastic process models are widely employed for analyzing spatial-temporal data in various scientific disciplines including, but not limited to, environmental monitoring, ecological systems, forestry, hydrology, meteorology, and public health. After estimating a spatial-temporal process for a given data set, inferential 12 interest may turn to estimating rates of change, or directional spatial gradients, over space and time. Dr. Banerjee’s research on Bayesian estimation of smoothness of spatial processes has led to fully modelbased inference for spatial and temporal gradients and the development of measures to quantify how gradients change along a spatial boundary. Dr. Banerjee’s contribution has been to offer, within a flexible spatial-temporal process setting, a framework to estimate arbitrary directional gradients over space at any given time-point, temporal derivatives at any given spatial location and, finally, mixed spatial-temporal gradients that reflect rapid change in spatial gradients over time and vice-versa. Drs. Banerjee and Gelfand are widely considered to be pioneers of this field, which is often referred to as wombling. A selection of five relevant publications is presented below.
1. Banerjee, S., Gelfand, A.E. and Sirmans, C.F. (2003). Directional rates of change under spatial process models. Journal of the American Statistical Association, 98, 946–954. DOI: http://dx.doi. org/10.1198/C16214503000000909
2. Banerjee, S. and Gelfand, A.E. (2006). Bayesian Wombling: Curvilinear gradient assessment under spatial process models. Journal of the American Statistical Association, 101, 1487–1501. DOI: http://dx.doi.org/10.1198/016214506000000041
3. Banerjee, S. (2010). Spatial gradients and wombling. In Handbook of Spatial Statistics, eds. A.E. Gelfand, P. Diggle, P. Guttorp, and M. Fuentes. Boca Raton, FL: Taylor and Francis/CRC, pp. 559– 574. DOI: http://dx.doi.org/10.1201/9781420072884-c31
4. Quick, H., Banerjee, S. and Carlin, B.P. (2015). Bayesian modeling and analysis for gradients in spatiotemporal processes. Biometrics, 71, 575–584. DOI: http://dx.doi.org/10.1111/biom.12305
5. Halder, A., Banerjee, S. and Dey, D.K. (in press). Bayesian modeling with spatial curvature processes. Journal of the American Statistical Association. DOI: https://doi.org/10.1080/01621459. 2023.2177166.
6.4 Modeling and inference for large, or massive, spatial-temporal data sets (“BIG DATA”):
Spatial process models for analyzing geostatistical data entail computations that become prohibitive as the number of spatial locations become large. Dr. Banerjee has led pioneering contributions in model-based solutions for spatial “BIG DATA” analysis. These include the development and implementation of classes of low-rank spatial process models known as “predictive processes” that achieve dimension-reduction by projecting the original process on an optimal lower-dimensional subspace. Another, more recent, line of research led by Dr. Banerjee develop sparsity-inducing spatial processes known as “Nearest-Neighbor Gaussian processes” (NNGP) that achieve scalabilty by exploiting sparsity in models without discarding spatial information. Finally, Dr. Banerjee has also explored “meta-kriging”, which refers to dividing and conquering massive spatial data sets by analyzing subsets of the data and subsequently pooling the analyses to draw inference for the entire data. These approaches have attracted significant attention among statisticians and practitioners and are widely deployed to deliver inference for massive spatial databases without compensating for richness of modeling. Five important publications in this domain include:
1. Banerjee, S., Gelfand, A.E., Finley, A.O. and Sang, H. (2008). Gaussian predictive process models for large spatial datasets. Journal of the Royal Statistical Society: Series B (Methodology), 70, 825– 848. DOI: http://dx.doi.org/10.1111/j.1467-9868.2008.00663.x
2. Datta, A., Banerjee, S., Finley, A.O. and Gelfand, A.E. (2016). Hierarchical nearest-neighbor Gaussian process models for large geostatistical datasets. Journal of the American Statistical Association, 111, 800–812. DOI: http://dx.doi.org/10.1080/01621459.2015.1044091
3. Banerjee, S. (2017). High-dimensional Bayesian geostatistics. Bayesian Analysis, 12, 583–614. DOI: http://dx.doi.org/10.1214/17-BA1056R.
4. Guhaniyogi, R. and Banerjee, S. (2018). Meta-Kriging: Scalable Bayesian modeling and inference for massive spatial datasets. Technometrics, 60, 430–444. DOI: https://doi.org/10.1080/00401706. 2018.1437474.
5. Peruzzi, M., Banerjee, S. and Finley, A.O. (2022). Highly scalable Bayesian geostatistical modeling via meshed Gaussian processes on partitioned domains. Journal of the American Statistical Association, 117, 969–982. DOI: https://doi.org/10.1080/01621459.2020.1833889
6.5 Disease mapping and spatial boundary analysis:
Regional aggregates of health outcomes over delineated administrative units (e.g., states, counties, and zip codes), or areal units, are widely used by epidemiologists to map mortality or incidence rates and capture geographic variation. Spatial dependence in such models are usually captured using graphical probability distributions such as Markov random fields that model the spatial effects conditionally given the effects of neighboring regions. Professor Banerjee has explored diverse aspects of such models and their inferential performance. He has developed novel classes of multivariate conditional auto-regression (MCAR) models and models building upon directed acyclic graphs (DAGAR models). He has also published original developments on spatial boundary analysis or areal wombling to identify neighboring regions with significant health oriented spatial disparities. Five selected publications in this field include:
1. Jin, X., Carlin B.P., and Banerjee, S. (2005). Generalized hierarchical multivariate CAR models for areal data. Biometrics, 61, 950–961. DOI: http://dx.doi.org/10.1111/j.1541-0420.2005.00359.x
2. Martinez-Beneito, M.A., Botella-Rocomara, P. and Banerjee, S. (2017). Towards a multi-dimensional approach to Bayesian disease mapping. Bayesian Analysis, 12, 239–259. DOI: http://dx.doi.org/ 10.1214/16-BA995
3. Li, P., Banerjee, S., Hanson, T.A. and McBean, A.M. (2015). Bayesian models for detecting difference boundaries in areal data. Statistica Sinica, 25, 385–402. DOI: http://dx.doi.org/10.5705/ss.2013.238w
4. Datta, A., Banerjee, S., Hodges, J.S. and Gao, L. (2019). Spatial disease mapping using directed acyclic graph auto-regressive (DAGAR) models. Bayesian Analysis, 14, 1221–1244. DOI: https://doi.org/10.1214/19-BA1177.
5. Gao, L., Banerjee, S. and Ritz, B. (in press). Spatial difference boundary detection for multiple outcomes using Bayesian disease mapping. Biostatistics. DOI: 10.1093/biostatistics/kxac013
6.6 Bayesian modeling and statistical learning from mechanistic systems:
Machine learning and high performance computing have unfurled conundrums surrounding the role of mechanistic modeling in scientific inference. Mechanistic systems refer to models built from scientific principles and laws that help understand complex mechanisms posited to be generating observational data. While machine learning algorithms often seek to circumvent the complex mechanisms through the use of large-scale data sets, mechanistic models attempt to directly relate the data to the laws of basic science. These two modeling paradigms often tend to operate exclusive to each other. Dr. Banerjee has developed approaches for integrating these two apparently antagonistic paradigms into a single comprehensive inferential framework. Building upon developments in Bayesian inferential frameworks for spatial-temporal 14 mechanistic models, Dr. Banerjee has proposed stochastic dynamical systems engendering full probabilistic uncertainty quantification for environmental processes. Five selected publications are:
1. Zhang, Y., Banerjee, S., Yang, R., Lungu, C. and Ramachandran, G. (2009). Bayesian modelling of air flow and exposure using two-zone models. Annals of Occupational Hygiene, 53, 409–424. DOI: http://dx.doi.org/10.1093/annhyg/mep017
2. Finley, A.O., Banerjee, S. and Basso, B. (2011). Improving crop model inference through Bayesian melding with spatially-varying parameters. Journal of Agricultural, Biological and Environmental Statistics, 16, 453–474. DOI: http://dx.doi.org/10.1007/s13253-011-0070-x
3. Monteiro, J.V., Banerjee, S. and Ramachandran, G. (2014). Bayesian modeling for physical processes in industrial hygiene using misaligned workplace data. Technometrics, 56, 238–247. DOI: http://dx.doi.org/10.1080/00401706.2013.836988
4. Datta, A., Banerjee, S., Finley, A.O., Hamm, N.A.S. and Schaap, M. (2016). Non-separable dynamic nearest neighbor Gaussian process models for large spatio-temporal data with application to particulate matter analysis. Annals of Applied Statistics, 10, 1286–1316. DOI: http://dx.doi.org/10.1214/16-AOAS931 (Winner of the 2017 American Statistical Association’s Outstanding Application Award).
5. Abdalla, N., Banerjee, S., Ramachandran, G. and Arnold, S. (2020). Bayesian state space modeling of physical processes in industrial hygiene. Technometrics, 62, 147–160. DOI: https://doi.org/10.1080/00401706.2019.1630009
6.7 Case studies requiring statistical innovation:
The advent of Geographical Information Systems and related technologies have led to a burgeoning of spatial databases in a diverse set of disciplines. Statisticians and spatial analysts often encounter large to massive spatial and spatial-temporal data sets that are incomplete, involve layers of complex dependencies and demand accurate assessment of uncertainty. Professor Banerjee has undertaken several such projects throughout his career in substantive scientific fields such as ecology, forestry, climate and the environment, and public health. Five significant publications here include:
1. Latimer, A.M., Banerjee, S., Sang, H., Mosher Jr., E. and Silander, J.A. (2009). Hierarchical models for spatial analysis of large data sets: A case study on invasive plant species in the northeastern United States. Ecology Letters, 12, 144–154. DOI: http://dx.doi.org/10.1111/j.1461-0248.2008.01270.x
2. Finley, A.O., Banerjee, S. and MacFarlane, D.W. (2011). A hierarchical model for predicting forest variables over large heterogeneous domains. Journal of the American Statistical Association, 106, 31–48. DOI: http://dx.doi.org/10.1198/jasa.2011.ap09653
3. Delamater, P.L., Finley, A.O. and Banerjee, S. (2012). An analysis of asthma hospitalizations, air pollution, and weather conditions in Los Angeles County, California. Science of the Total Environment. 425, 110–118. DOI: http://dx.doi.org/10.1016/j.scitotenv.2012.02.015
4. Adgate, J.L., Banerjee, S., Wang, M., McKenzie, L.M., Hwang, J., Cho, S.J. and Ramachandran, G. (2013). Performance of dust allergen carpet samplers in controlled laboratory studies. Journal of Exposure Science and Environmental Epidemiology, 23, 385–391. DOI: http://dx.doi.org/10.1038/jes.2012.112
5. Foster, J.R., Finley, A.O., D’Amato, A.W., Bradford, J.B. and Banerjee, S. (2016). Predicting tree biomass growth in the temperate-boreal ecotone: Is tree size, age, competition or climate response most important? Global Change Biology, 22, 2138–2151. DOI: http://dx.doi.org/10.1111/gcb.13208
6.8 Computational methods, algorithms and statistical software development:
Spatial data analysis for massive data sets with complex dependencies often require specialized computational algorithms in conjunction with scalable models. Professor Banerjee devotes considerable efforts in efficient algorithms and implementation of Bayesian hierarchical models for spatial and space-time data sets. Most of these enterprises are co-authored with his former doctoral students who have produced software to accompany the methodological developments in their dissertations. Five selected publications related to Bayesian computational methods, algorithms and software development are:
1. Ren, Q., Banerjee, S., Finley, A.O. and Hodges, J.S. (2011). Variational Bayesian methods for spatial data analysis. Computational Statistics and Data Analysis, 55, 3197–3217. DOI: http://dx.doi.org/10.1016/j.csda.2011.05.021
2. Eidsvik, J., Finley, A.O., Banerjee, S. and Rue, H. (2012). Approximate Bayesian inference for large spatial datasets using predictive process models. Computational Statistics and Data Analysis, 56, 1362–1380. DOI: http://dx.doi.org/10.1016/j.csda.2011.10.022
3. Finley, A.O., Banerjee, S. and Gelfand, A.E. (2015). spBayes: for large univariate and multivariate point-referenced spatio-temporal data models. Journal of Statistical Software, 64, 1–28. DOI: http://dx.doi.org/10.18637/jss.v063.i13
4. Finley, A.O., Datta, A., Cook, B.C., Morton, D.C. Andersen, H.E. and Banerjee, S. (2019). Efficient algorithms for Bayesian nearest-neighbor Gaussian processes. Journal of Computational and Graphical Statistics, 28, 401–414. DOI: https://doi.org/10.1080/10618600.2018.1537924.
5. Zhang, L., Datta, A. and Banerjee, S. (2019). Practical Bayesian modeling and inference for massive spatial datasets on modest computing environments. Statistical Analysis and Data Mining: The ASA Data Science Journal, 12, 197–209. DOI: https://doi.org/10.1002/sam.11413
6.9 Impact and relevance of scholarly research:
Professor Banerjee’s Google Scholar Citation indices are presented in Table 3. The methods and inferential frameworks he has developed are widely adopted by practicing data scientists and researchers investigating space-time data. His first-authored manuscript d Banerjee et al. (JRSS-B, 2008) developed a very popular spatial-temporal dimension-reducing stochastic process called the “Gaussian predictive process”, while his paper Datta et al (JASA; 2016) where he is preceded by his doctoral student Abhi Datta as first author developed a class of “Nearest-Neighbor Gaussian Processes” (NNGP) for truly massive spatial-temporal databases with tens of millions of space-time coordinates. The former has attracted over 1,135 citations, while the NNGP paper (currently with upward of 500 citations) consistently ranked among the Journal of the American Statistical Association’s five most-cited articles within 5 years of its publications and was cited accompanying Professor Banerjee’s George W. Snedecor Award in 2019. Both these methods are widely employed to analyze large to massive data sets in diverse fields including climate science, ecology, econometrics, environmental sciences, forestry, epidemiology and kernel-based machine learning. A subsequent manuscript from the final chapter of Datta’s dissertation (Datta et al., 2016; Annals of Applied Statistics) mapped environmental pollution levels in continental Europe at unprecedentedly large space-time resolutions and fetched the authors an Outstanding Application Award from the American Statistical Association.
Professor Banerjee has over 22 manuscripts on original statistical methodologies as a leading author that have attracted over 100 citations with most of the aforementioned articles under spatial survival analysis being among the first in that domain. His more theoretical papers on properties of spatial estimators and features of spatial processes have also been widely used by practitioners. For example, Banerjee, 2005; Biometrics explored the impact of using geodesic distance computations on erstwhile statistical practices for spatial data analysis, while a recent theoretical treatise from doctoral and postdoctoral supervision Tang et al., 2021; JRSS-B on the effects of delineating white noise from spatial signals is attracting increasing attention in the spatial data science and machine learning communities.
6.10 Textbooks and Monographs
Professor Banerjee has co-authored 2 textbooks as leading author. Hierarchical Modeling and Analysis for Spatial Data (with Gelfand and Carlin) is in its second edition and is considered an authoritative textbook on spatial statistics (with over 4220 citations). His second textbook, Linear Algebra and Matrix Analysis for Statistics (with Dr. A. Roy), was published in 2015 and is widely appreciated for its clarity of presentation making the subject accessible to statisticians. In addition, he is one of four editing authors for the Handbook of Spatial Epidemiology published in 2016, which is an authoritative reference on the use of spatial data science in epidemiology and biostatistics.